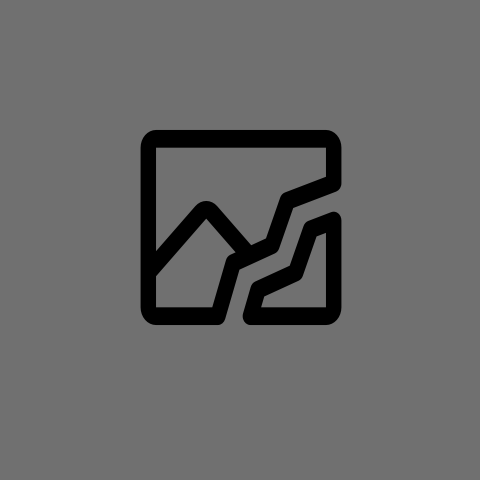
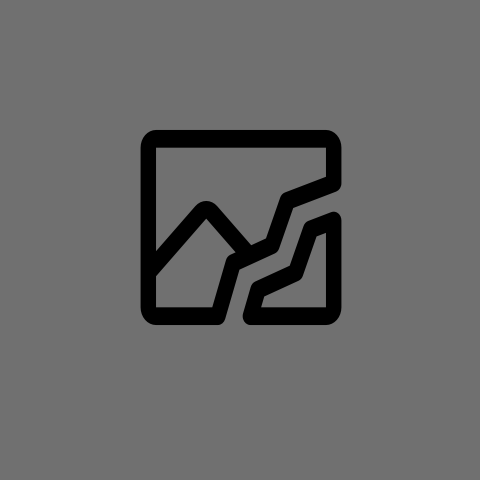
People haven’t really changed. As always, power corrupts. When the rewards are great enough, it seems people are often enough willing to compromise their integrity.
People haven’t really changed. As always, power corrupts. When the rewards are great enough, it seems people are often enough willing to compromise their integrity.
I have LTS and zen kernels installed in addition to the default Arch one, that should prevent this yes?
KDE Connect and Syncthing do the trick for most stuff. For all else, all hail the USB C M.2 NVME enclosure.
Note the versions, none of the results give you the official operators page for the current version, 16. They give 9, which went EOL in 2021.
Have you tried recent models? They’re not perfect no, but they can usually get you most of the way there if not all the way. If you know how to structure the problem and prompt, granted.
Them using Google indexes anonymously isn’t intending to solve the problem you think it is. It’s more about incentive structures. Google’s “free” search optimizes for ad revenue now. The API access doesn’t as much, and Kagi certainly doesn’t have an ad incentive. So privacy is a nice bonus, but the real benefit is a customer serving incentive structure.
Funny how the DOS equivalent of ls is dir, so before the GUI folder metaphor.
A major caveat I’ve noticed some people misunderstand: it’s corporate CLAs that are problematic. The Apache Foundation also requires contributors sign a CLA, but it’s to provide a legal fail safe and a way to update to say Apache 3.0 if need be one day. Apache’s non profit, open source mission aligns with respecting the rights of contributors and the community. Corporations, on the other hand, not so much.
Codeberg is run off of donations, they have no service contract revenue. Nobody, much less a volunteer, wants to commit to a 5 or 10 year service plan like that, it’s not sustainable for a small project from a non profit.
CLAs can be abusive, but not necessarily. Apache Foundation contributors need to sign CLAs, which essentially codify in contract form the terms of the Apache 2.0 license. It’s a precaution, in case some jurisdiction doesn’t uphold the passive licensing scheme used otherwise. There’s also a relicensing clause, but that’s restricted to keeping in spirit, they can’t close the source.
I use Kagi, they provide access to all the main models in a chat interface and have a mode that feeds search engine results to them. It’s mostly replaced search engines for me. For programming work I find them very useful for using unfamiliar tools and libraries, I can ask it what I want to so and it’ll generally tell me how correctly. Importantly, the search engine mode has citations. $25 a month, but worth it.
The idea that “it’s ok cause we’d do the same” is ridiculous. There is no comparison: China is an authoritarian government and the parent company is practically an arm of the state. There are legitimate criticisms of American tech companies obviously, but they’re ultimately subject to the market and democratic governments. We shouldn’t be doing any business with authoritarians in the first place, much less inviting them to control a significant social media app in the guise of a legitimate business.
It runs great now. Most importantly, it supports extensions like ublock.
So this is probably another example of Google using too blunt of instruments for AI. LLMs are very suggestible and leading questions can severely bias responses. Most people using them without knowing a lot about the field will ask “bad” questions. So it likely has instructions to avoid “which is better” and instead provide pros and cons for the user to consider themselves.
Edit: I don’t mean to excuse, just explain. If anything, the implication is that Google rushed it out after attempting to slap bandaids on serious problems. OpenAI and Anthropic, for example, have talked about how alignment training and human adjustment takes a majority of the development time. Since Google is in a self described emergency mode, cutting that process short seems a likely explanation.
Arch for stuff I have physical access to. Nothing’s ever gone wrong, so it’s worth it for the immediate updates and consistency with my other systems. For VPS I use Debian though, occasionally the unstable/Sid branch if I really need the latest updates. There are almost always Debian images available on a VPS.
If I’m understanding this right, and this basically an API that lets you pick which app store administers an app, that could be quite helpful, not harmful. I currently have fdroid, play store, and Samsung store, and I assume they try to update apps by the fully qualified name, as multiple stores show and try to update a single app instance, sometimes with weird results.
Compression is actually a mathematical field that’s fairly well explored, and this isn’t compression. There are theoretical limits on how much you can compress data, so the data is always somewhere, either in the dictionary or the input. Trained models like these are gigantic, so even if it was perfect recall the ratio still wouldn’t be good. Lossy “compression” is another issue entirely, more of an engineering problem of determining how much data you can throw out while making acceptable compromises.
This is a classic problem for machine learning systems, sometimes called over fitting or memorization. By analogy, it’s the difference between knowing how to do multiplication vs just memorizing the times tables. With enough training data and large enough storage AI can feign higher “intelligence”, and that is demonstrably what’s going on here. It’s a spectrum as well. In theory, nearly identical recall is undesirable, and there are known ways of shifting away from that end of the spectrum. Literal AI 101 content.
Edit: I don’t mean to say that machine learning as a technique has problems, I mean that implementations of machine learning can run into these problems. And no, I wouldn’t describe these as being intelligent any more than a chess algorithm is intelligent. They just have a much more broad problem space and the natural language processing leads us to anthropomorphize it.
Normal people don’t, but when you get into absolutely massive enterprise archiving there’s no rival for the density and cost effectiveness. It sucks for general purpose storage, but for write once, hopefully never read use, they’re ideal.
I recently removed in editor AI cause I noticed I was acquiring muscle memory for my brain, not thinking through the rest past the start of a snippet that would get an LLM to auto complete. I’m still using LLMs, particularly for languages and libraries I’m not familiar with, but using the artifacts editors in ChatGPT and Claude.