
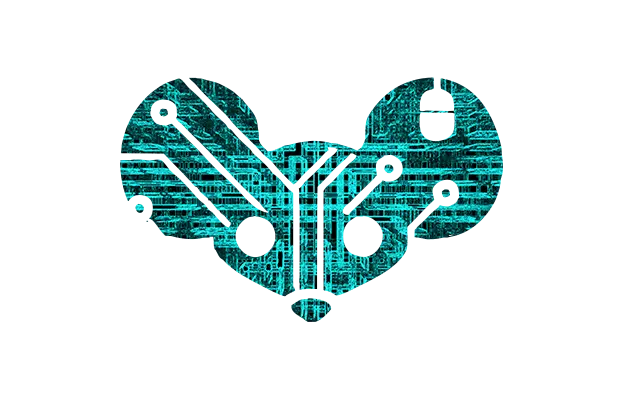
By the same logic, raytracing is ancient tech that should be abandoned.
Nice straw man argument you have there.
I’ll restate, since my point didn’t seem to come across. All of the “AI” garbage that is getting jammed into everything is merely scaled up from what has been before. Scaling up is not advancement. A possible analogy would be automobiles in the late 60s and 90s: Just put in more cubic inches and bigger chassis! More power from more displacement does not mean more advanced. Continuing that analogy, 2.0L engines cranking out 400ft-lb and 500HP while delivering 28MPG average is advanced engineering. Right now, the software and hardware running LLMs are just MOAR cubic inches. We haven’t come up with more advanced data structures.
These types of solutions can have a place and can produce something adjacent to the desired results. We make great use of expert systems constantly within narrow domains. Camera autofocus systems leap to mind. When “fuzzy logic” autofocus was introduced, it was a boon to photography. Another example of narrow-ish domain ML software is medical decision support software, which I developed in a previous job in the early 2000s. There was nothing advanced about most of it; the data structures used were developed in the 50s by a medical doctor from Columbia University (Larry Weed: https://en.wikipedia.org/wiki/Lawrence_Weed). The advanced part was the computer language he also developed for quantifying medical knowledge. Any computer with enough storage, RAM, and the hardware ability to quickly traverse the data structures can be made to appear advanced when fed with enough collated data, i.e. turning data into information.
Since I never had the chance to try it out myself, how was your neural network and LLMs reasoning back in the day? Imo that’s the most impressive part, not that it can write.
It was slick for the time. It obviously wasn’t an LLM per se, but both were a form of LM. The OCR and auto-suggest for DOS were pretty shit-hot for x386. The two together inspried one of my huge projects in engineering school: a whole-book scanner* that removed page curl and gutter shadow, and then generated a text-under-image PDF. By training the software on a large body of varied physical books and retentively combing over the OCR output and retraining, the results approached what one would see in the modern suite that now comes with your scanner. I only achieved my results because I had unfettered use of a quad Xeon beast in the college library where I worked. That software drove the early digitization processes for this (which I also built): http://digitallib.oit.edu/digital/collection/kwl/search
*in contrast to most book scanning at the time, which required the book to be cut apart and the pages fed into an automatically fed scanner; lots of books couldn’t be damaged like that.
Edit: a word
And an additional response, because I didn’t fully answer your question. LLMs don’t reason. They traverse a data structure based on weightings relative to the occurrence frequency in their training content. Loosely speaking, it’s a graph (https://en.wikipedia.org/wiki/Graph_(abstract_data_type)). It appears like reasoning because the LLM is iterating over material that has been previously reasoned out. An LLM can’t reason through a problem that it hasn’t previously seen unlike, say, a squirrel.